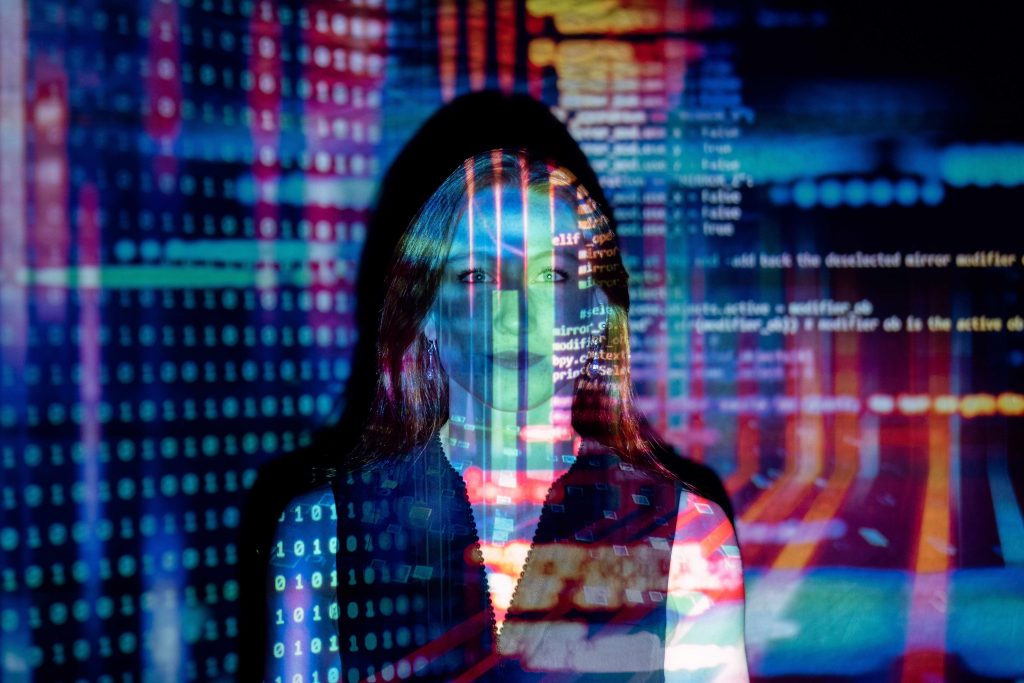
According to many people, artificial intelligence, or AI, is a sort of cure-all for modern organisations’ woes. However, one of the biggest mistakes that you can make when adopting AI is assuming that it’s a silver bullet that will magically catapult your firm to the forefront of your industry. Rather than having these astronomical expectations for its capabilities, it’s better to have a more grounded and realistic view. we’ve found that the best ways to do so are by shifting your view about AI; by thinking about capturing information in a strategic manner; and by making the use of AI technology truly useful and convenient for your customers.
1. Change your Mindset
The 21st century that was envisioned by the popular cartoon show The Jetsons, society would rely on robots like Rosie, the robotic, automated maid that could do anything and everything for the family. These robots would be relegated to completely handling a variety of repetitive, monotonous tasks. In doing so, they would ostensibly free us up to enjoy our lives more fully.
What we are already learning, however, is that progress is actually made more quickly when we design and build such “robots” to instead tackle very small, specific – and often seemingly trivial – tasks. Instead of designing robotic servants that take care of a huge variety of daily needs, we are finding that it’s better to create programs that focus on mastering much smaller things. In fact, this is the very basis for the concept of machine learning, which plays a major role in helping us to realise the full potential of AI for business.
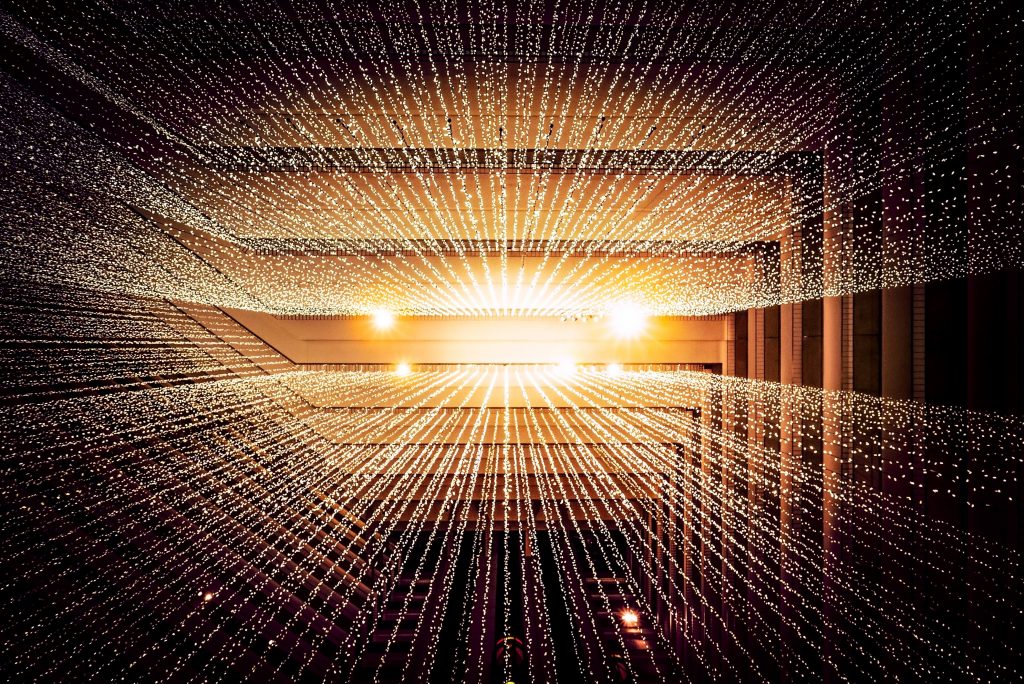
Consider this: When the washing machine was first invented, its sole purpose was to agitate dirty clothes in soapy water to shift that burden from humans to machines. Over time, insights regarding such machines’ potential capabilities were gleaned through the collection of customer feedback and other data. In turn, we have been able to improve these “robots” so that they can accomplish specific tasks – like sensing the types of clothes being washed and how dirty they are – that vastly improve their overall functionality and usefulness.
Each algorithm covers only one small aspect of what an intelligent machine like Rosie The Robot could do.
When this mindset is applied to AI – improving overall outcomes through the gradual refinement of smaller, simpler tasks – machine learning kicks in. In turn, the true potential of everything from data science to AI is more easily realized. What I’m trying to say here is that AI doesn’t handle “doing it all” very well. Where it really shines is in focusing on proficiency with smaller, tightly focused, seemingly trivial tasks. When a series of such small tasks is put together, the resulting machine comes the closest that it can hope to achieving true “intelligence.”
Let me put this another way: Despite all of the hype, AI is flat-out dumb. This is an extremely valuable perspective for putting AI into effective business use. Don’t expect for any given AI solution to magically resolve a major issue or to achieve a critical objective in one fell swoop. Understand that despite its big promise, AI really isn’t a silver bullet – and then go from there.
Where to go, though, exactly? As with the gradual refinements that have been made with washing machine technology, it’s best to focus first on small, even seemingly trivial tasks. Once your AI has achieved proficiency with several specific tasks, they can be strung together. This Frankenstein’s monster of sorts will then appear to be highly intelligent. It won’t actually be, of course – but it will definitely seem that way.
There is no “one” AI, but a number of algorithms that work in unicent to pretend to show “intelligence”.
So it’s not a problem that each algorithm possesses the approximate intelligence of a microbe. Instead, be satisfied with teaching it to achieve small, simple steps to solve one small very specific problem. It will do great in that. Then refine each of those steps as you gather more information and feedback, and then refine them further. Finally, put them all together to create something that helps you to realise the full, exciting potential of AI.
2. Capture Strategic Data, In Automated Ways
Contrary to what serves as conventional wisdom in many – if not most – industries, quantity isn’t the be-all, end-all when it comes to information. Rather, the quality of that information is what really matters. In fact, having copious amounts of data usually means that a lot of that data is of little or no value to your organisation. In many industries – financial services, telecommunications and healthcare, for instance – it’s easy to quickly accumulate vast amounts of digital data, but that doesn’t mean that it does you an iota of actual good.
In these types of industries, such information may be gathered and saved for periods of 10 years or longer. Unfortunately, however, this historical data won’t necessarily help with the things that matter like predicting customer behaviour or optimising decision making across an organisation.
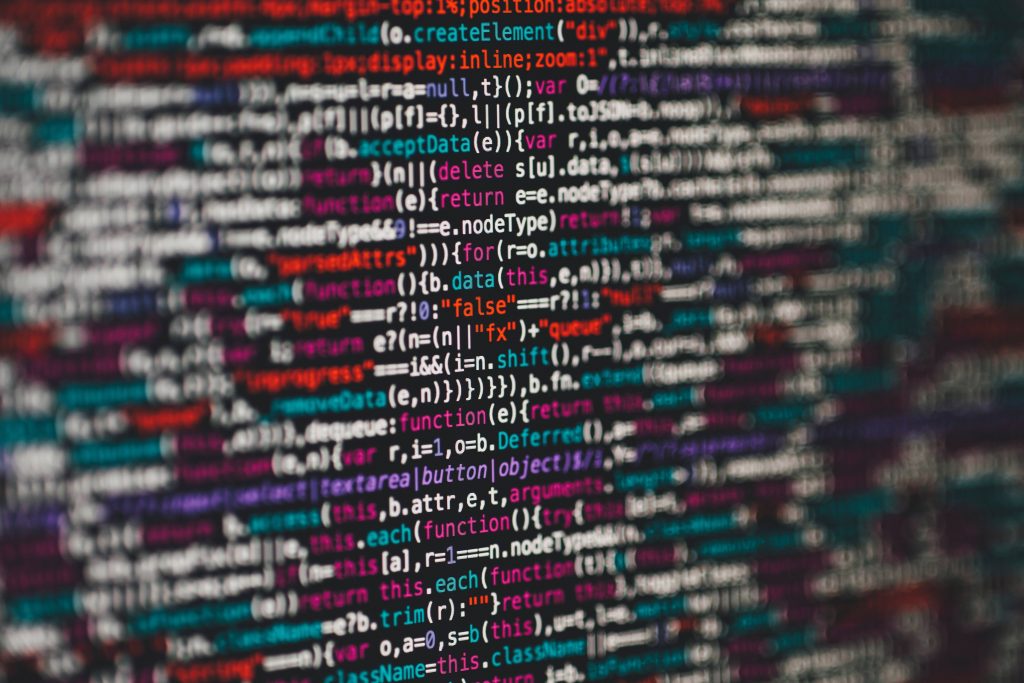
Various reasons may come into play to compromise such historical information, including:
- Frequent shifts in the regulatory landscape
- The ongoing evolution of systems that capture and store information
- The introduction of new competitors
- The evolution of new technologies
- Changes in customer preferences and expectations along with many more
For this reason, data scientists should play a crucial role in the ways your company collects information that is used for AI purposes. After all, modern systems often code the same pieces of data into different categories. The manner in which information is entered often shifts too – for example, updates in training protocols are inevitable, and employees often find and use various loopholes.
Data scientists should play a crucial role in the ways your company collects information that is used for AI purposes.
How to resolve this, though? Wherever possible, automated information capturing and automated information verification methods should be introduced. However, it’s crucial to understand that such techniques are hardly silver bullets, either. Even with such safeguards, it’s easy to end up with low-quality information that’s riddled with errors. You can also easily wind up with information that’s missing entirely or that is labeled or categorised in ways that do little or nothing to bolster the effects of AI.
This is where effective data science and analytics come into play – or should. The best way to employ this strategy is by working with an experienced data scientist. Such professionals work to define information that will be useful for each specific project and help you see the pitfalls that lie ahead. In doing so, they vastly improve the quality of the information that is gathered. Because when it comes to information for AI, quality really does usually trump quantity.
Still, if casting a wide net and getting as much data as possible isn’t the way to go when gathering information, how are you supposed to accumulate unique assets that help you to realise the potential of AI? Don’t worry: There are still plenty of opportunities for doing so; few and specific data points may be enough to give you a unique competitive advantage. This may be on their own or in combination with others.
Few relevant data points that are the appropriate fuel your algorithm are much more valuable than a sea of data that’s of mixed quality and low yield.
A great way to capitalise on this concept is by starting very small with your information capturing efforts, especially if you’re doing something new. Although the sum total of what you gather may be small, it will also be completely new and unique. This will then allow you to develop the world’s largest repository of information that’s pertinent to that specific pain point or issue that your product is trying to address. The dataset may be tiny, but it could also still be the biggest on the planet for your particular niche.
Case in point: Our team once built a dataset for the mining industry through the text processing of journal papers, industry articles and technical reports. Our AI processed tens of thousands of documents. It compiled information from the 40 largest underground mines at the time, measuring and comparing them on 120 variables over a timespan of about 100 years or so. The resulting dataset was hardly massive, but it was extremely useful to mining companies, mining equipment manufacturers and other key industry players.
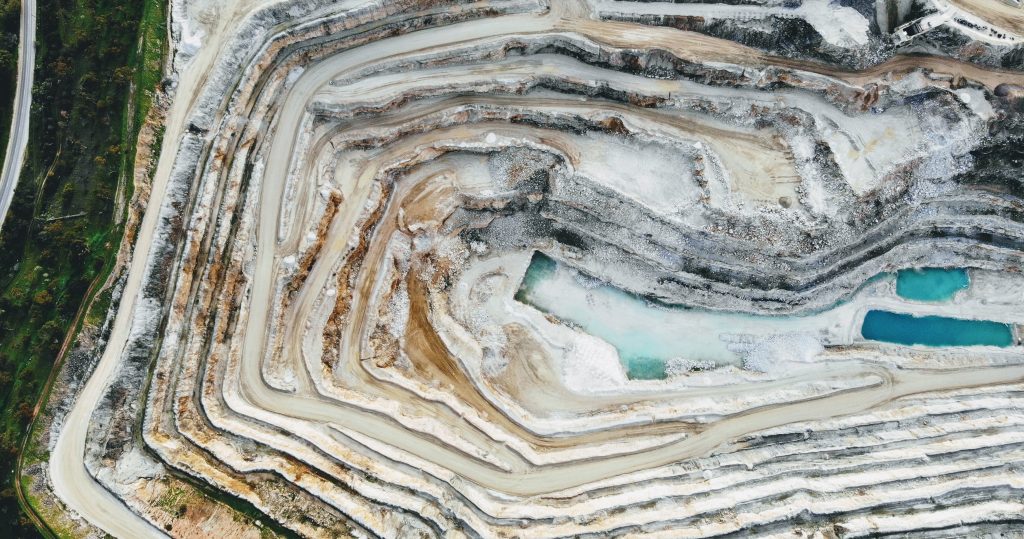
3. Make It Convenient and Beneficial for Your Customers
Thirdly, you can’t realise the full value of AI by benefiting your company only. It should also be useful and convenient for your customers. We can look at modern automobile sensors for a great analogy of how this concept can work in AI. The sensors in your car help to make technologies like adaptive cruise control possible – and those technologies provide direct benefits for end users.
You can’t realise the full value of AI by benefiting your company only.
Through the use of sensor technology, modern vehicles now offer features that provide immediate benefits for customers in the form of improved safety, for example. Because these advances are so beneficial to customers, they value these technologies and are even happy to pay extra for them. In this way, customers don’t just benefit from this technology – automobile manufacturers do too.
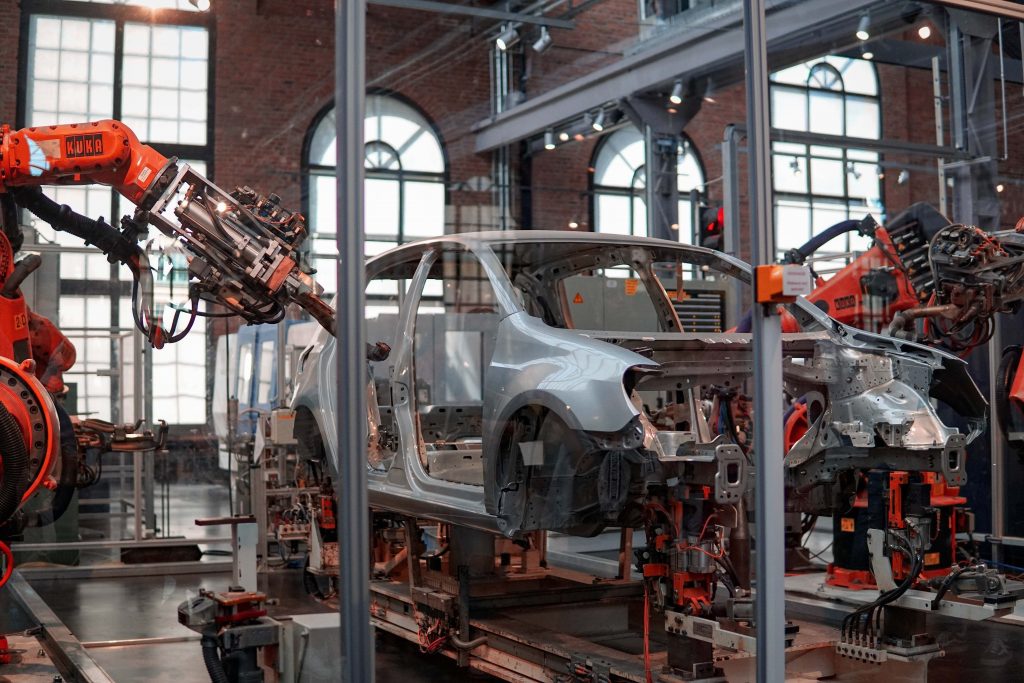
What would you think if your car had those same sensors installed, but the information that they collected was only shown to manufacturers with no benefit to the customer? That would be pretty creepy, right? If nothing else, it would leave a bad taste in your mouth; you’d feel like you were being spied on, almost. You certainly wouldn’t be enthusiastic about paying extra for such technology. After all, it wouldn’t directly benefit you in any discernible way, so why would you be?
The positive impact of things like AI and its ongoing adoption by the public comes not from catering to your organisation’s needs – at least not solely – but from catering to those of your customers. Therefore, when adopting and employing AI for business, you should always ask yourself how any particular technology will prove to be beneficial to end users. How likely will customers be to not just adopt such advances but to do so enthusiastically? Will they be happy to absorb the cost of developing such innovations, or would they scoff at the very idea?
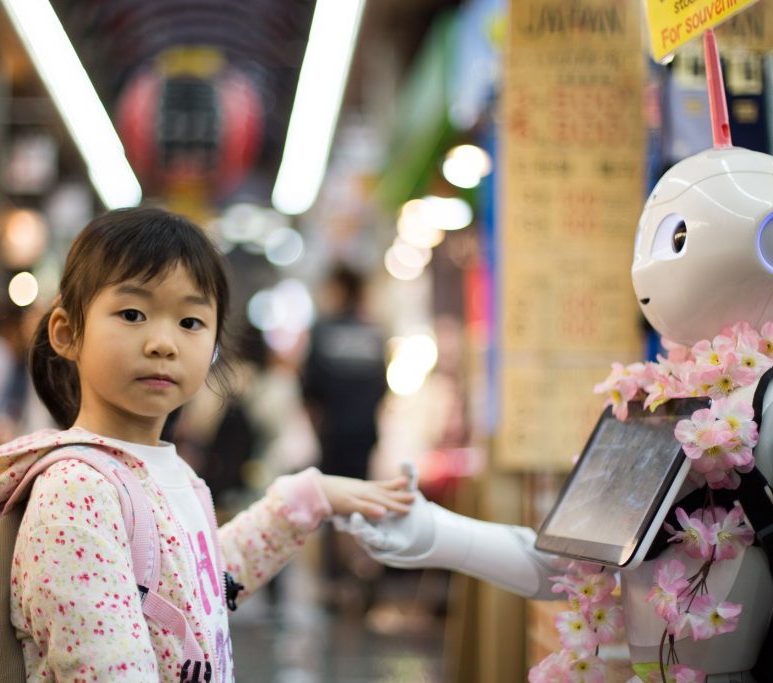
Just as technological innovations in vehicles have been developed slowly and progressively over time, the AI technologies that you use can – and probably should – be developed gradually. The important thing isn’t rushing to push out new innovations in AI but in ensuring that those innovations are in line with the interests of your target audience. When they are – even if they are very incremental – they will more readily be adopted by customers. In turn, it will be easier to continually improve upon them to achieve the maximum potential from your AI and machine learning efforts.
With these points in mind, remember that it’s perfectly fine to start in an incredibly small and simple way when developing AI for your business. In fact, it is probably always in your best interests to do so. Since technology advances at such a breakneck speed, it’s easy to assume that your adoption, development and use of AI should too. However, doing so will leave your customers in the lurch. In the end, it will stymy your efforts to make the most of AI and will leave you wondering why you bothered with it in the first place.
Given what they have already done with sensors and other forms of AI technology in modern vehicles, we have some pretty good ideas about where car manufacturers will go next with the information that they gather from them. In particular, we believe that they will use that data to provide you with insights about your driving that you can then use to be safer and more conscientious behind the wheel. For example, your car might alert you that you do your most dangerous driving on Wednesdays between the hours of 8 p.m. and 9 p.m. Ideally, this alert would come through as you are getting into your car to head back home at, say, 8:27 p.m., allowing you to keep that information in mind as you drive off.
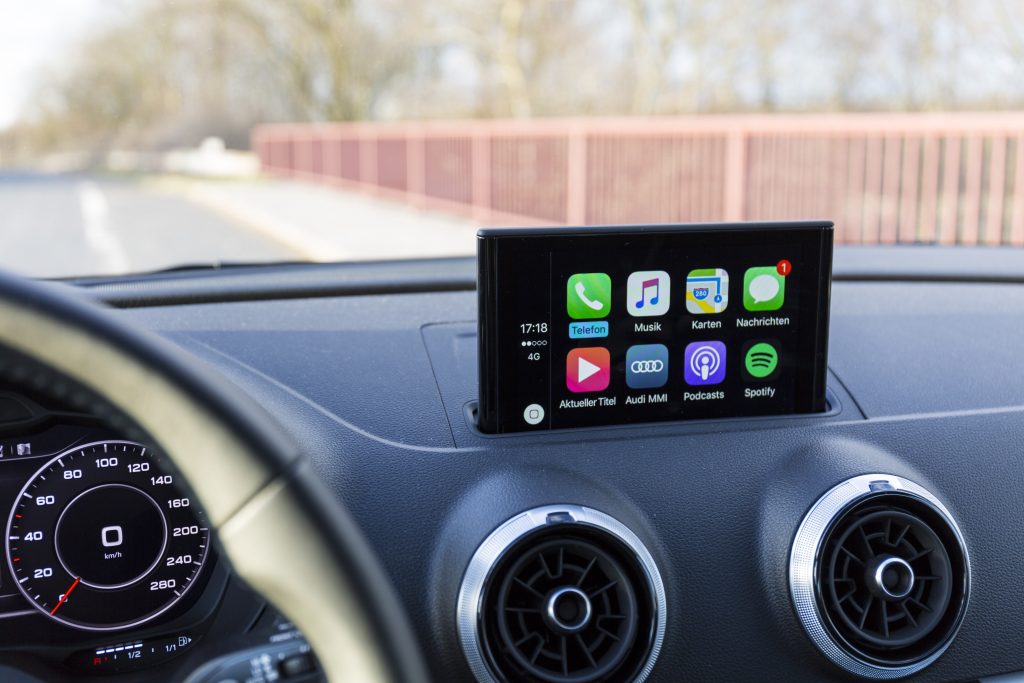
The current missed opportunity for car manufacturers here is that they are not providing insights to the drivers based on the information these sensors are capturing. Imagine if they did. How valuable would that be for you? What similar opportunities to use AI for the benefit of your customers are you missing in your organisation?
Just as car companies can continually reap the benefits of the information that they gather through AI technologies, your organisation can too. However, for that to happen, you’ll need to approach the situation effectively. The best way to do that is by keeping the above three points in mind every step of the way. Therefore, focus on shifting your mindset: AI is dumb. Make capturing information strategically a priority: Data science matters. Finally, work on making that technology convenient and beneficial for customers: Starting small and simple is the way to go.
For more data insights like this, follow us on LinkedIn and tune in to our partners at Data Futurology for their world leading data podcasts.
Have a data problem that needs solving? Reach out to the RUBIX team today.